Project overview:
Gene activity is regulated in a coordinated manner. With the knowledge that many functionally related genes collectively contribute to a biological process, the analysis can be shifted from individual genes to gene sets resulting in significant reduction of noise and greater biological interpretability. Furthermore, it enhances the statistical power of test of association of phenotype with genetic variants by pooling signals of set of genes linked to same biological process. Despite the decade long research into the field, some of the most popular gene set analysis methods still suffer from serious limitations in specificity, P-value calculation methods, stability of scoring functions, and applicability to wide range of biological datasets. The goal of this project is develop novel efficient statistical methods for gene set analysis addressing the current limitations and apply them for highthroughput data analysis.
Latest methods:
mGSZm (2016) : We have developed a gene set analysis method, mGSZm, for multi-group gene expression dataset with as few as three replicates per group. Our method has shown the best performance as compared to the state-of-the-art gene set analysis methods with three different real gene expression datasets and evaluation methods.
mGSZ (2014) : mGSZ is a gene set analysis method based on Gene Set Z-score (Törönen et al.,2009) and asymptotic P-value. mGSZ is different from the conventional gene set analysis methods in that it assigns asymptotic P-values to the gene set scores instead of empirical P-values. Asymptotic p-value calculation involves fitting a suitable asymptotic model to the null distribution of gene set scores. Asymptotic P-value calculation requires considerably fewer permutations as compared to the empirical P-value calculation to accurately estimate P-values and thus speeds up the gene set analysis process.
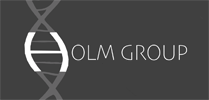
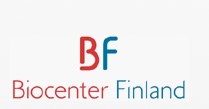
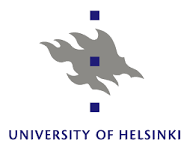